Have you ever found yourself at the edge of your seat, biting your nails as a cricket match unfolds, and wondered, “How to Calculate the Predicted Score in Cricket?” Whether you’re a die-hard fan, a strategic coach, or someone trying to make informed bets, knowing how to calculate the predicted score in cricket can enhance your game experience. In this article, we’ll unravel the complexities behind score prediction, diving into various factors and methods experts use to forecast cricket match outcomes.
Understanding Cricket Scores
Cricket scores are a tapestry of numbers and statistics. At its core, the score reflects runs scored by the batting team and wickets taken by the bowling team. But predicting the score isn’t just about adding up these numbers; it’s about understanding the story they tell.
- Runs: The total number of runs scored by a team.
- Wickets: The number of batters dismissed.
- Overs: The number of completed overs, giving insight into the pace of scoring.
Each of these elements intertwines to create the final score; understanding them is the first step in predicting it.
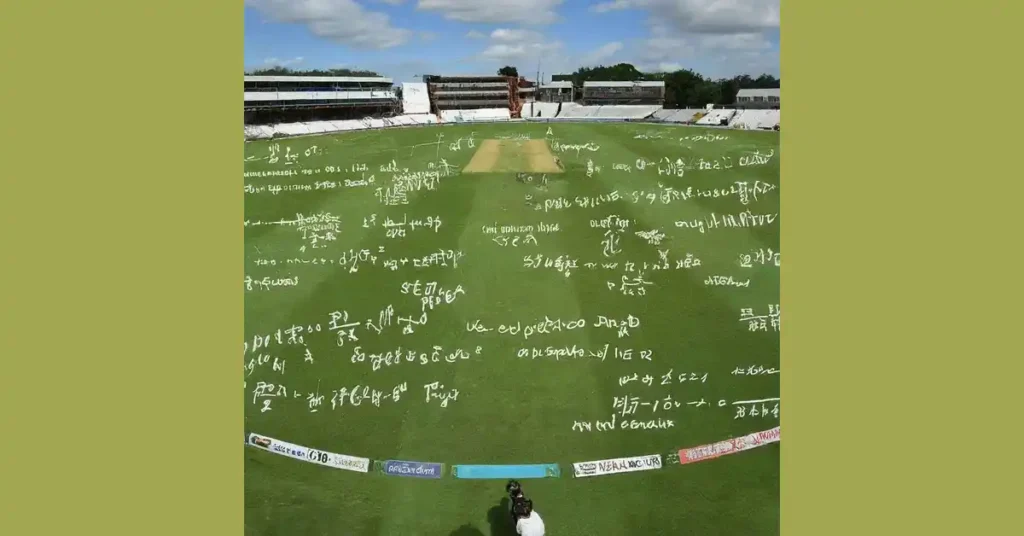
Importance of Score Prediction
Why bother with score prediction? Well, it goes beyond just satisfying curiosity. Accurate score predictions can:
- Enhance Viewing Experience: Knowing potential outcomes makes the match more engaging.
- Strategic Planning: Coaches and players can devise better game strategies.
- Betting Insights: Bettors can make more informed decisions, increasing their chances of winning.
- Analytical Skills: Develop a deeper understanding of the game, turning you into a cricket connoisseur.
Critical Factors in Score Prediction
Several factors come into play when predicting cricket scores. Let’s break down the most crucial ones.
1. Cricket Pitch Analysis
The pitch is the stage where the cricket drama unfolds. Its condition can significantly influence the match outcome. Here’s how:
- Dry Pitch: Favors spin bowlers, making it challenging for batters.
- Green Pitch: This benefits fast bowlers, leading to low-scoring games.
- Flat Pitch: A batting paradise, often resulting in high scores.
Understanding pitch conditions is crucial for accurate score predictions.
2. Cricket Weather Conditions
Weather isn’t just a backdrop in cricket; it’s a game-changer. Different weather conditions can tilt the game for either batsmen or bowlers.
- Sunny: Generally better for batters, with fewer interruptions.
- Overcast: Helps swing bowlers, making batting more challenging.
- Rainy: This can lead to reduced overs, affecting score projections.
Checking weather forecasts is a vital step in predicting cricket scores.
3. Team Batting and Bowling Strengths
Every team has unique strengths and weaknesses, which can sway the final score.
- Strong Batting Line-up: Teams with powerful hitters and consistent performers tend to score higher.
- Robust Bowling Attack: Teams with skilled bowlers can restrict the opposition to lower scores.
Analyzing these strengths can provide insights into how a match might unfold.
4. Player Performance History
Past performance is often a good predictor of future success. Here’s what to consider:
- Batting Averages: Higher averages indicate reliable run-scorers.
- Bowling Averages: Lower averages suggest effective bowlers.
- Recent Form: Current performance trends can hint at future results.
Looking at player statistics helps in making informed score predictions.
5. Head-to-Head Team Records
Historical data of previous encounters between teams can be very telling.
- Dominance: If one team consistently outperforms another, it will likely continue.
- Close Matches: Frequent nail-biters suggest a closely fought game.
By understanding and analyzing these factors, you can forecast cricket scores more accurately. Whether it’s the pitch conditions, weather, team strengths, player form, or historical records, each element plays a vital role in the art and science of cricket score prediction. With this knowledge, you can elevate your cricket-watching experience, make strategic decisions, and even outwit the bookmakers. So, next time you watch a match, put your new skills to the test and see if you can predict the score like a pro!
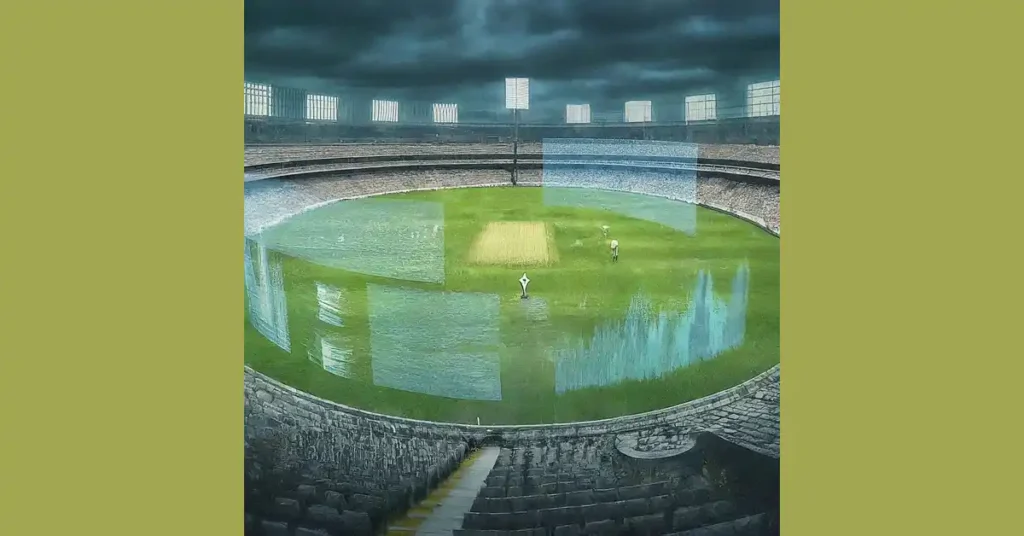
Prediction Methods
Predicting cricket scores involves various methods, each with unique approaches and tools. Let’s explore some of the prominent methods used for this purpose.
1. Cricket Match Prediction Algorithms
Algorithms designed for cricket match prediction analyze vast amounts of data to forecast match outcomes. These algorithms consider historical match data, player statistics, and game conditions. By processing this data, they generate predictions about the likely scores and outcomes of matches. Some popular algorithms include:
- Naïve Bayes: Utilizes probability theory to predict outcomes based on historical data.
- Decision Trees: Classifies data into different categories to predict outcomes.
- Support Vector Machines (SVMs): Analyzes data to find the best classification boundaries for predicting match results.
2. Statistical Analysis for Cricket Score Prediction
Statistical analysis involves examining historical match data to identify trends and patterns. This method uses various statistical models to estimate probable scores. Key elements include:
- Player Averages: Batting and bowling averages provide insights into expected performance.
- Run Rates: Historical run rates help predict future scoring patterns.
- Match Conditions: Factoring in pitch, weather, and venue statistics to refine predictions.
3. Cricket Score Prediction Tools
Many online tools and software are designed to predict cricket scores. These tools use data from past matches and current forms to generate predictions. Examples include:
- Cricket Prediction API: Provides real-time predictions using machine learning models.
- Win Predictor Tools: Estimate each team’s probability of winning based on live match data and historical trends.
- Score Simulators: Simulate possible match scenarios to predict scores and outcomes.
4. Machine Learning in Cricket Score Prediction
Machine learning has revolutionized cricket score prediction by enabling models to learn from vast datasets and improve over time. These models can handle complex patterns and make accurate predictions based on numerous variables. Key techniques include:
- Supervised Learning: Trains models on labeled data to predict outcomes.
- Unsupervised Learning: Identifies hidden patterns in data without predefined labels.
- Reinforcement Learning: Improves prediction models by learning from feedback and adjusting strategies accordingly.
5. Cricket Betting Odds Analysis
Betting odds provide valuable insights into score prediction. Bookmakers use sophisticated models to set odds, reflecting the probability of various outcomes. By analyzing these odds, one can gauge:
- Market Sentiment: Indicates how the betting market views the match outcome.
- Odds Movements: Changes in odds can suggest shifting probabilities based on new information (e.g., player injuries, weather updates).
- Value Bets: Identifying discrepancies between predicted outcomes and betting odds to find profitable opportunities.
Specific Scoring Factors
Several specific scoring factors play a critical role in predicting cricket scores. Understanding these factors can significantly enhance the accuracy of predictions.
1. Cricket Batting Average
The batting average is a fundamental statistic that measures a batsman’s performance. It is determined by dividing the total number of runs scored by the number of times the batter has been removed. A higher batting average indicates a more consistent and reliable batter.
2. Cricket Bowling Average
The bowling average is a crucial metric for evaluating a bowler’s effectiveness. It is computed by dividing the total number of runs allowed by the number of wickets taken. A lower bowling average suggests a more efficient and impactful bowler, often leading to lower scores for the opposition.
3. Cricket Win/Loss Ratio
The win/loss ratio measures a team’s overall performance. It is determined by dividing the number of matches won by the number of lost matches. A higher win/loss ratio indicates that a more robust team will perform well in future matches, influencing score predictions.
4. Cricket Run Rate
The run rate, or the rate at which runs are scored, is crucial for understanding a team’s scoring potential. You calculate it by dividing the total runs scored by the number of overs faced. A higher run rate indicates a more aggressive and effective batting performance, leading to higher predicted scores.
5. Cricket Strike Rate
The strike rate measures how quickly a batsman scores runs and is calculated by dividing the total number of runs by the number of balls faced, then multiplying by 100. For bowlers, it is the number of balls bowled per wicket taken. Higher batting strike rates suggest more aggressive scoring, while lower bowling strike rates indicate more frequent wicket-taking.
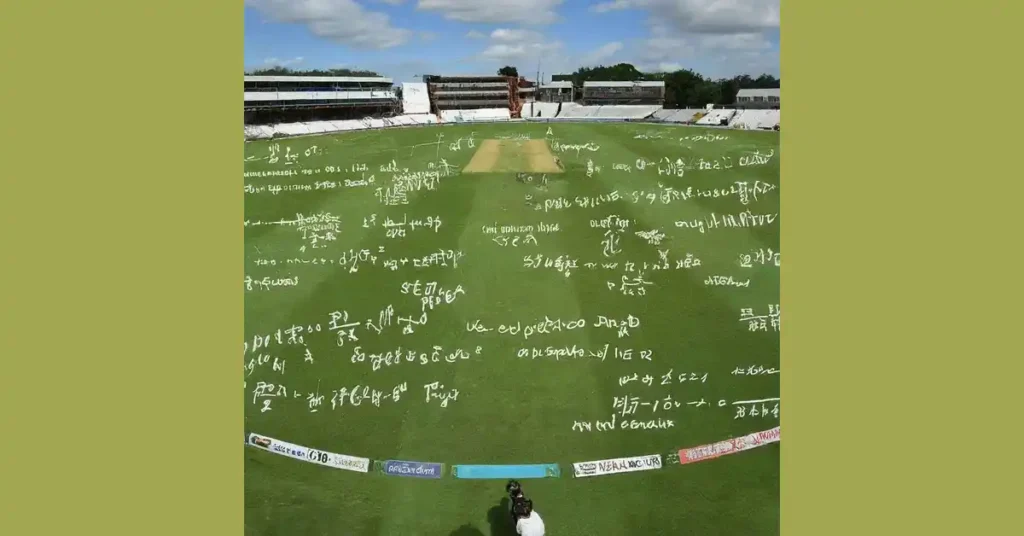
Developing a Prediction Model
Creating a robust prediction model for cricket scores involves several steps:
- Data Collection: Gather historical data on matches, including scores, player statistics, weather conditions, and pitch reports.
- Data Preprocessing: Clean the data to handle missing values and standardize the format.
- Feature Selection: Identify critical variables influencing the outcome, such as batting averages, strike rates, and win/loss ratios.
- Model Selection: Based on the complexity and nature of the data, choose an appropriate machine learning algorithm (e.g., linear regression, decision trees, or neural networks).
- Training and Testing: Dividing the data into training and testing sets will help you determine the model’s effectiveness.
- Validation: Use cross-validation techniques to ensure the model’s accuracy and generalizability.
- Deployment: Implement the model in a real-world setting to make predictions and continuously update it with new data for improved accuracy.
Case Study: Example Match Prediction
To show this, let’s look at a case study of guessing how an ODI cricket match will end:
- Data Collection: Historical match data, including team performance, individual player stats, and environmental factors.
- Feature Selection: Key features like team batting strength, bowling averages, and past head-to-head records are selected.
- Model Selection: A logistic regression model is chosen for its simplicity and effectiveness in binary outcomes.
- Training and Testing: 80% of the data are used to teach the model, and the other 20% are used to test it.
- Evaluation: The model’s accuracy is assessed using precision, recall, and F1-score metrics.
Following these steps, the prediction model can accurately forecast match outcomes, providing valuable insights for teams and analysts.
Conclusion: How to Calculate the Predicted Score in Cricket?
To accurately calculate the predicted score in cricket, you need to know many things, like how well the players are doing, how the teams work together, and the weather. Using modern statistical techniques and algorithms for machine learning, it is possible to make accurate prediction models that make planning strategies and decisions in cricket much easier.
->What Type Of Bet Is More Profitable?
->How Do You Study Betting Lines? A Complete Guide
Player performance history and team dynamics are crucial factors.
Weather conditions can significantly influence pitch behavior and player performance, affecting score predictions.
Algorithms for machine learning can look through many data to find trends and make predictions.
Batting and bowling averages provide insights into player consistency and effectiveness, which is crucial for predicting scores.
Models should continuously update with new data to maintain accuracy and relevance.